An Empirical Study of Model Errors and User Error Discovery and Repair Strategies in Natural Language Database Queries
Published at
IUI
| Syndey, Australia
2023
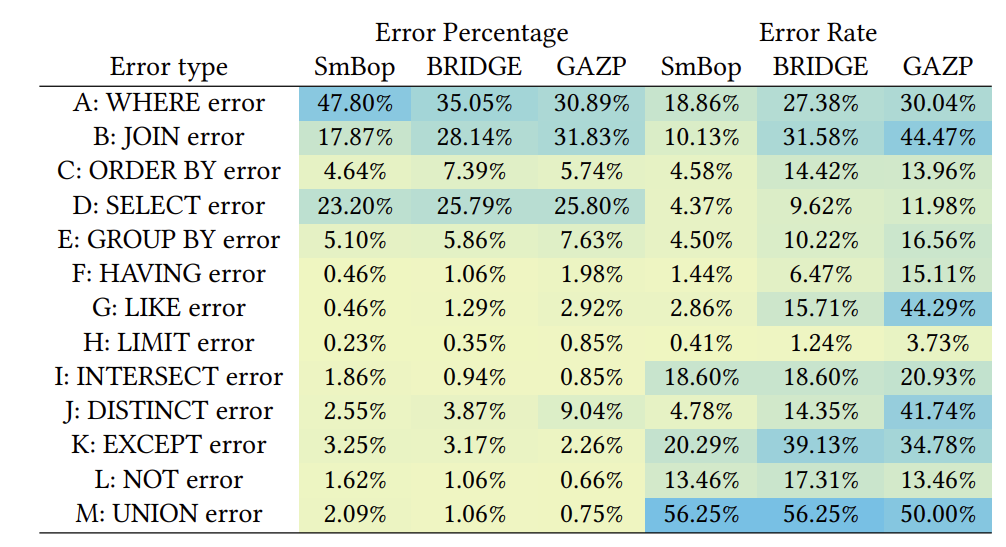
Abstract
Recent advances in machine learning (ML) and natural language
processing (NLP) have led to significant improvement in natural
language interfaces for structured databases (NL2SQL). Despite
the great strides, the overall accuracy of NL2SQL models is still far
from being perfect (∼75% on the Spider benchmark). In practice, this
requires users to discern incorrect SQL queries generated by a model
and manually fix them when using NL2SQL models. Currently, there
is a lack of comprehensive understanding about the common errors
in auto-generated SQLs and the effective strategies to recognize
and fix such errors. To bridge the gap, we (1) performed an in-depth
analysis of errors made by three state-of-the-art NL2SQL models;
(2) distilled a taxonomy of NL2SQL model errors; and (3) conducted
a within-subjects user study with 26 participants to investigate the
effectiveness of three representative interactive mechanisms for
error discovery and repair in NL2SQL. Findings from this paper shed
light on the design of future error discovery and repair strategies
for natural language data query interfaces.